Molecular docking and 3D-QSAR studies of 2-substituted 1-indanone derivatives as acetylcholinesterase inhibitors1
Introduction
Alzheimer’s disease (AD) is a progressive and neuro-degenerative disorder of the brain, with a loss of memory and cognition, which and is a common form of dementia among the elderly[1]. Acetylcholinesterase (AChE), one of the most essential enzymes in the family of serine hydrolases, catalyzes the hydrolysis of neurotransmitter acetylcholine, which plays a key role in memory and cognition. It is clear that the cholinergic deficiency is associated with AD[2], therefore, one of the major therapeutic strategies for the treatment of AD is to inhibit the biological activity of AChE, and hence to increase the acetylcholine level in the brain. Currently, most of the drugs used in clinics for the treatment of AD are AChE inhibitors, such as donepezil and rivastig-mine, which have been proven to improve the situation of AD patients to some extent.
Four drugs have been approved by the Food and Drug Administration (FDA) to treat AD in the US so far, namely tacrine, rivastigmine, donepezil, and galanthamine. In addition, huperzine A was marketed in China for the same purpose, but only as a dietary supplement in the US. The 3-D structure of AChE from native Torpedo californica (TcAChE) has been determined by X-ray crystallography (Protein Data Bank, PDB code: 2ACE)[3]. There are also a lot of complex structures of TcAChE with inhibitors determined experi-mentally, such as donepezil (PDB code: 1EVE)[4], tacrine (PDB code: 1ACJ)[5], decamethonium ion (PDB code: 1ACL)[5], m-(N,N,N-trimethylammonio) trifluoroacetophen-one (PDB code: 1AMN)[6], huperzine A (PDB code: 1VOT)[3], galanthamine (PDB code: 1DX6)[7], and edrophonium (PDB code: 2ACK)[8]. All these structures significantly enhance our understanding of the structural elements of AChE. The binding pocket of AChE is a long and narrow region which consists of 2 separated ligand binding sites: the catalytic (central) site and the peripheral anionic site[3,4,6]. The catalytic site is the binding site of classical AChE inhibitors, such as tacrine and huperzine A, which has been studied thoroughly. On the contrary, the function of the peripheral site has not been elucidated clearly as yet. Recent studies have demonstrated that the peripheral site might accelerate the aggregation and deposition of beta-amyloid peptide, which is considered as another cause of AD[2,9,10]. Therefore, it is supposed that an ideal AChE inhibitor should bind to the catalytic and peripheral sites simultaneously, which could disrupt the interactions between the enzyme and the beta-amyloid peptide, and hence slow down the progression of the disease[11,12].
Among the AChE inhibitors, donepezil and rivastigmine are usually used early to moderate the stages of AD patients to treat cognitive loss. However, neither of them could cure AD, and they often cause some adverse effects. For example, donepezil can lead to diarrhea, and rivastigmine can cause vomiting. Therefore, it is urgent to find more effective AChE inhibitors to treat AD[11,12]. From the crystal structure, it is clear that the 5,6-dimethoxy-1-indanone moiety and benzyl-piperidine moiety of donepezil interact with the peripheral and catalytic binding site of AChE, respectively[4], whereas rivastigmine is the catalytic site-binding inhibitor[13]. Therefore, combining the characteristics of the 2 inhibitors, Sheng et al recently reported a new kind of AChE inhibitors 2-substituted 5,6-dimethoxy-1-indanone derivatives, which have exhibited excellent inhibitory activities on AChE in vitro[14,15]. These compounds consist of 3 functional groups: a 5,6-dimethoxy-1-indanone, a benzene ring, and a protonated nitrogen (Table 1). The first moiety, extracted from donepezil, is supposed to interact with the peripheral binding site, while the other 2 moieties, derived from rivastigmine (Table 1), might be able to interact with the catalytic binding site.
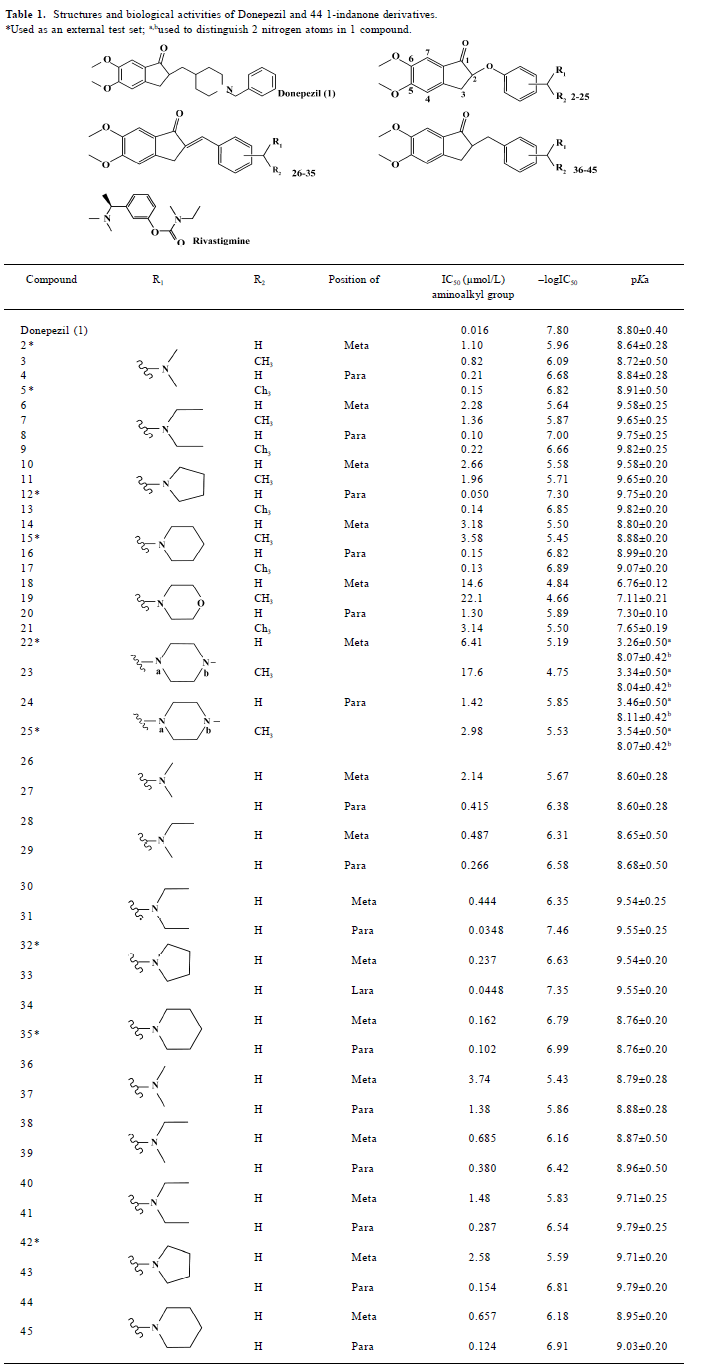
Full table
Recently, Akula et al performed molecular docking and comparative molecular field analysis (CoMFA) on a series of bis-tacrine compounds, which provides some new clues for the rational design of AChE inhibitors[16]. In order to further elucidate the mechanism of these 1-indanone derivatives on the enzyme and provide hints for a new derivative design, molecular docking and three-dimensional quantitative structure-activity relationship (3D-QSAR) studies were carried out in the present study. At first, 44 1-indanone derivatives, together with donepezil, were docked into the active site of the enzyme, and then the detailed interactions between the inhibitors and the enzyme were investigated. Based on the docked conformations of these compounds within the active site of AChE, the 3D-QSAR analyses were performed directly using both CoMFA and comparative molecular similarity analysis (CoMSIA) methods. The CoMFA and CoMSIA contour plots were subsequently mapped onto the active site of the enzyme, which provided more information for the structural modification of this kind of inhibitors.
Materials and methods
Preparation of ligands We modeled the structures of 44 1-indanone derivatives synthesized by Sheng et al[14,15]. Their structures and bioactivities, together with donepezil, totally 45 compounds are listed in Table 1. Nine compounds (com-pounds with asterisks in Table 1) were randomly selected as an external test set for further model validation, and the rest of the 36 compounds served as a training set to build the 3D-QSAR models. The 3-D structures of these compounds were sketched with molecular modeling software package SYBYL version 7.0 (Tripos Associates, St Louis, Missouri, USA)[17] and energetically minimized using the Tripos force field[18] with Gasteiger-Hückel charges[19]. According to the inhibitors’ pKa values[20] (Table 1), the nitrogen atoms of ligands 1–21 and 26–45 were protonated. In the cases of ligands 22–25, only the nitrogen adjacent to the methyl group was protonated since its pKa value was more than 7 and the other nitrogen’s pKa value was much less than 7. All the calculations were performed on a Dell Precision 670 workstation running Redhat Linux WS 3.0 (Red Hat, North Carolina, USA)
Preparation of the enzyme The crystal structure of TcAChE in the complex with donepezil was used in the study[4]. The ionization states of some residues in TcAChE were determined with the method of Gilson et al[21]. The residues His440, Glu443, and Asp392 were neutralized as pointed out by McCammon et al[22]. Because the pharmacological activities of the inhibitors were determined in rat cortex homo-genates, the enzyme should be rat AChE instead of TcAChE. The only difference between rat AChE and TcAChE in the binding site was that Phe330 of TcAChE was replaced by Tyr330 of rat AChE, therefore Phe330 was mutated to Tyr330. All the other ionizable residues were kept in their standard protonation states. A few tightly bound water molecules in the active site (WAT1158, WAT1159, WAT1160, WAT1161, WAT1249, and WAT1254) were considered in the docking of donepezil because they played a key role in ligand binding[4], but they were removed when the other 44 compounds were docked into the enzyme. After adding all the hydrogen atoms, the donepezil–TcAChE complex was relaxed for 400 steps using the Tripos force field[18] with Kollman all-atom charges[23] for the enzyme and Gasteiger–Hückel charges[19] for the ligand in SYBYL.
Molecular docking GOLD version 3.0.1 (Cambridge Crystallographic Data Centre, Cambridge, UK)[24] was employed to investigate the binding mode between the inhibitors and AChE. The binding site of TcAChE was defined as all the residues within 17 Å from the CE1 atom of Phe331. The default parameters of genetic algorithms (GA) were applied to search the reasonable binding conformation of these flexible ligands. In order to find more accurate geometries, the option “allow early termination” was not selected. The maximum number of GA runs was set to 10 for each compound. The ChemScore[25] fitness function was used to evaluate the docking conformations as proposed by Guo et al[26]. Those docked conformations were saved in MOL2 format and then imported into SYBYL for further analysis. The donepezil pose derived from GOLD was used as the reference compound for alignment. The final conformation for each ligand was selected based on the following criteria: at first considering the conformer with the highest ChemScore[25], then considering the conformer forming a good π–π interaction between the indanone ring and Trp279, and lastly considering hydrogen bonding between the carbonyl group and the NH group of Phe288.
3D-QSAR analyses The docking geometries of all the compounds were aligned together within the binding pocket of TcAChE, which was directly used for further CoMFA[27] and CoMSIA[28] analyses to discuss the specific contributions of steric, electrostatic, hydrophobic, and hydrogen bond effects on the bioactivities of the inhibitors.
CoMFA steric and electrostatic field energies were probed using an sp3 carbon atom and a +1 net charge atom, respec-tively. The Tripos force field[18], with a distance-dependent dielectric constant at all intersections in a regularly spaced (2 Å) grid, had been set for the calculation of steric and electrostatic field interactions. The column-filtering threshold value was set to 2.0 kcal/mol to improve the signal-to-noise ratio. The default value of 30 kcal/mol was adopted as the maximum steric and electrostatic energy cut-off. The regression analysis was carried out using the full cross-validated partial least-squares (PLS) method (leave-1-out) with CoMFA standard options. The final conventional model was developed with the optimum number of components equal to that yielding the highest q2LOO. Predictive r2 (r2pred) values were calculated using the following equation: r2pred=(SD-PRESS)/SD, where PRESS is the sum of the squared deviation between the observed and predicted activities of the test molecules, and SD is the sum of the squared deviation between the biological activity of the test set and the mean activity of the training set.
To further validate the model, the leave-4-out cross-validation was applied to prove its robustness. In the leave-4-out cross-validation, all the compounds were first randomly divided into 9 groups with equal numbers of chemicals in each group. Each group was systematically excluded once from the data set when rebuilding the model. This procedure was repeated 100 times due to the random nature of the selection of chemicals in the process. The mean q2LNO and the standard deviation of q2LNO were reported to assess the model’s stability of prediction for diverse chemicals.
The 5 CoMSIA similarity index fields, namely steric, electrostatic, hydrophobic, hydrogen bond donor, and acceptor, respectively, were calculated at the grid points using a probe atom (Wprobe,,k) with charge of +1. The attenuation factor α was set to 0.3 for the Gaussian type distance. The statistical evaluation for the CoMSIA analyses was executed in the same way as described for CoMFA.
Mapping 3D-QSAR contours onto the AChE active site The CoMFA and CoMSIA results were visualized by coefficient contour maps. Compound 31 was displayed on the map in aid of visualization. The contour maps obtained from the CoMFA and CoMSIA models depicted actual versus fitted activities of the training set. Key residues within 4.0 Å around the ligand were visualized for further interpretations.
Results
Interactions of inhibitors with AChE To determine which docking method was suitable for the AChE inhibitors, a comparison of GOLD with FlexX was carried out on the enzyme in our previous work[29]. The results demonstrated that GOLD could reproduce the X-ray determined conformations of known AChE inhibitors very well. Therefore, the GOLD method was used in this study, and the resulting enzyme–inhibitor interaction was reliable.
In order to consider the influence of cocrystallized water molecules on docking, donepezil was docked into the binding pocket of TcAChE with and without water molecules, separately (Figure 1). The docking conformations of donepezil superimposed with the X-ray crystal structure very well, which indicated that the cocrystallized water had little impact on docking.
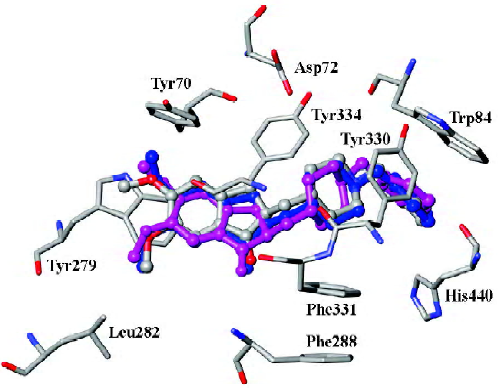
Our result illustrated the docking conformations of all 45 compounds aligned in the binding pocket of AChE (Figure 2). The 1-indanone structures superimposed with each other very well (Figure 2). The CoMFA and CoMSIA analyses were then performed based on the binding conformations and their alignments.
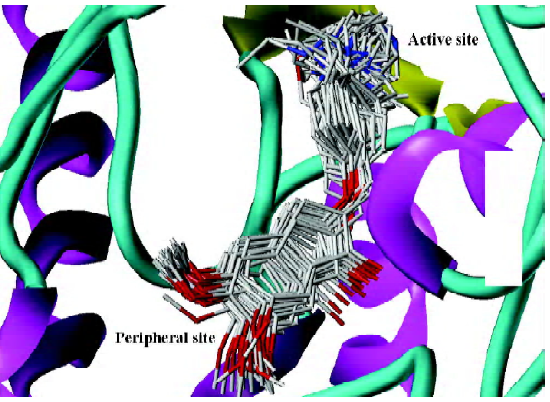
As mentioned above, compound 31, the most potent inhibitor among the 2-substituted 1-indanone derivatives, was selected as an example to illustrate the detailed interactions between inhibitors and the enzyme. As discussed later, all descriptions referred to compound 31, unless otherwise specified. Figure 3 shows the binding mode of compound 31 with AChE. In general, these inhibitors could be divided into 3 parts: the 5,6-dimethoxy-1-indanone moiety, the benzene ring moiety, and the protonated nitrogen moiety[30]. All the inhibitors formed major interactions with the active site of the enzyme through the 3 parts[31].
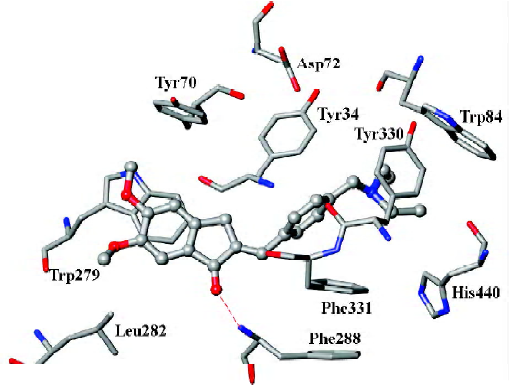
The 5,6-dimethoxy-1-indanone moiety of all the compounds was approximately located at the same site. It was obvious to see that the 5,6-dimethoxy-indanone moiety was surrounded by residues Tyr70, Leu282, and Trp279 through hydrophobic interactions at the entrance of the gorge (Figure 3). Among them, the indanone group formed a π–π stacking interaction with the indole ring of Trp279, while the 5,6-dimethoxy group interacted with the side chains of Leu282 and Trp279 through the hydrophobic interaction. The carbonyl group of the 5,6-dimethoxy-1-indanone moiety as an acceptor formed a hydrogen bond with the NH group of Phe288.
In the middle of the gorge, the region was very narrow and hydrophobic. The benzene ring of the inhibitor interacted with the aromatic rings of Tyr330, Phe331, and Phe334. Near the bottom of the gorge, the protonated nitrogen of the diethylamine group as an anchor, formed cation-π interactions with the aromatic planes of Trp84 and Tyr330. The diethylamine group could also form interactions with the side chain of His440.
3D-QSAR models
CoMFA The aim of the CoMFA analysis of these donepezil analogues was to find the best predictive model within the system. Thirty six of the 45 inhibitors were randomly picked up as the training set to build the CoMFA model. The remaining 9 were used as an external test set for the model validation. The results of the CoMFA analysis (leave-1-out) are summarized in Table 2 and Table 3. The PLS analysis (leave-1-out) gave a correlation with a cross-validated q2LOO of 0.784 and an optimum component number of 5. The non-cross-validated PLS analysis was repeated with 5 components, as determined by the cross-validated analysis, to give a conventional r2 of 0.974. These values indicated a good conventional statistical correlation. The predicted activities (PA) of the 45 inhibitors from the 3D-QSAR model versus their experimental activities (EA) are shown in Table 4, and the correlation between PA and EA is presented in Figure 4A.
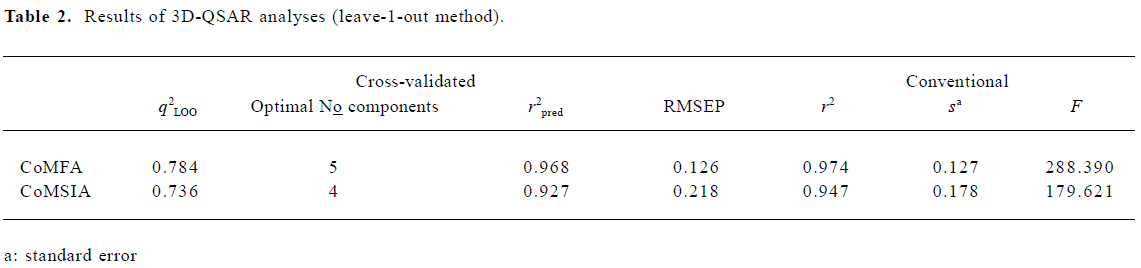
Full table
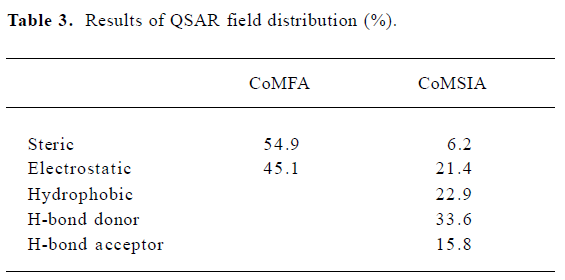
Full table
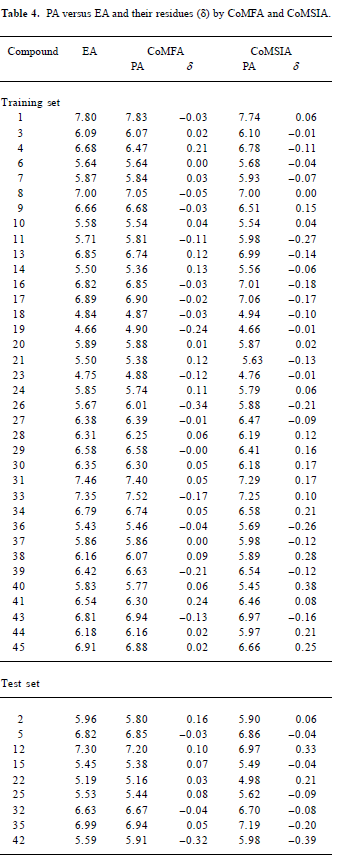
Full table
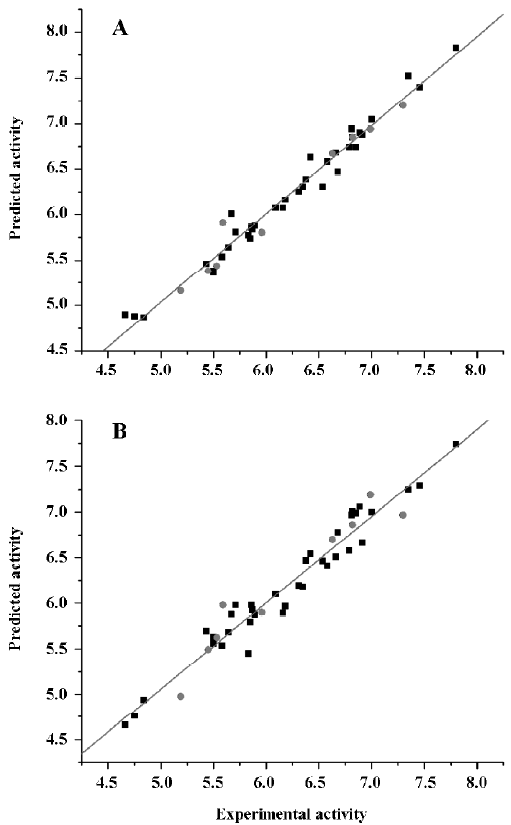
One important issue in QSAR is cross-validation, especially when the compounds in the test set are very similar to those in the training test. It has been shown that a high q2LOO is the necessary, but not the sufficient condition for the model to have a high predictive power[32]. Compared to the leave-1-out procedure, the leave-N-out procedure allows N compounds to be omitted for the prediction of the model’s stability. Therefore, q2LNO accounts for more extrapolation of the model than q2LOO does. Because of the random nature of the selection of chemicals in the leave-N-out process, the q2LNO is varied for a selected N group in each run. In order to obtain a valid statistical analysis, it is necessary to run the leave-N-out procedure several times (100 times in the present study) for each random N group. Consequently, the standard deviation of q2LNO can be used to assess the model’s stability for the bioactivity prediction of other compounds[33]. As an alternative validation, the leave-4-out method has been conducted for the CoMFA model. The leave-4-out cross-validated q2 value and standard deviation were 0.753 and 0.017, respectively, which provided a good measure of the statistical significance of the model again.
CoMSIA The CoMSIA analysis results (leave-1-out) are also summarized in Table 2 and Table 3. A CoMSIA model with a cross-validated q2LOO of 0.736 for 4 components and a conventional r2 of 0.947 was obtained. These data demonstrated that the constructed CoMSIA model was reliable. The predicted activities of the 45 inhibitors from the 3D-QSAR model versus their experimental activities are listed in Table 4 and their correlation is shown in Figure 4B. The high value of the conventional r2 relating to 5 different descriptor variables (steric, electrostatic, hydrophobic, hydrogen bond donor, and acceptor) illustrated that these variables were necessary to describe the interaction mode of the inhibitors with AChE, as well as the field properties around the inhibitors.
The leave-4-out method was also applied to the CoMSIA model and gave a cross-validated q2 value and a standard deviation of 0.723 and 0.019, respectively.
External validation of the 3D-QSAR models The external test set, composed of the 9 randomly selected inhibitors, was used to further validate the established 3D-QSAR models. The predicted activities of these compounds are shown in Figure 4 (red dots). It is easy to see from Figure 4 that the predicted –logIC50 values of the test compounds were in agreement with the corresponding experimental data, leading to predictive r2 values of 0.968 for CoMFA and 0.927 for CoMSIA. The root mean square error of prediction (RMSEP) in both models were 0.126 and 0.218, respectively. The results indicated that the constructed CoMFA and CoMSIA models could be used in the design of new 1-indanone derivatives.
Discussion
Comparison of binding mode of donepezil with those of other inhibitors In the crystal structure of the donepezil-TcAChE complex, the charged nitrogen of the piperidine ring made a cation–π interaction with the phenyl ring of Tyr330, while in the binding mode of compound 31 with AChE, the phenyl ring might have formed π–π interactions with the side chains of Tyr330, Phe331, and Phe334. The distance between the OD2 atom of Asp72 and protonated nitrogen in donepezil and compound 31 was 5.69 and 6.51 Å, respec-tively, which explained why donepezil was more active than compound 31.
The benzene ring in donepezil could form a π–π interaction with the side chain of Trp84. After the benzene ring was replaced with a positive-charged chain or cyclic amine in the new compounds, the protonated nitrogen formed a cation–π interaction with the side chains of Trp84 and Tyr330; the carbon chain adjacent to the protonated nitrogen could form hydrophobic interactions with the side chains of Trp84 and Tyr330, which meant that the interaction modes of new compounds were different from that observed in donepezil.
Mapping CoMFA and CoMSIA contours onto the AChE binding site Based on the above 3D-QSAR models, the CoMFA coefficient isocontour maps were made onto the active site of the enzyme in Figure 5. Compound 31 was still used as a reference molecule in the map. Colored polyhedra in the map indicated these areas in the 3-D space where changes in the field values for 1-indanone derivatives correlated strongly with concomitant changes in inhibitory activities.
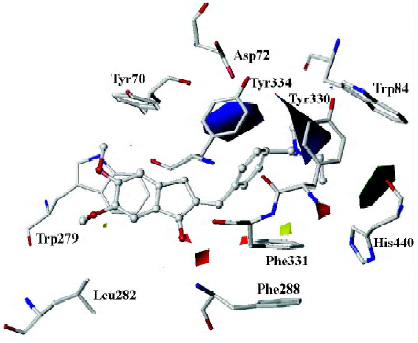
Steric and electrostatic interactions from CoMFA and CoMSIA In the CoMFA contour map, the green region around the R1 group suggested that a bulky substituent in the position might be favorable for biological activity, since many functional groups, such as the diethylamine group, pyrrolidine group, and piperidine group could interact with the side chains of Trp84, Tyr330, and His440 through the Van der Waals forces. However, if the R1 group is too large, it will be detrimental to the biological activity of the compound due to the possible clash between the compound and the active site of AChE. The yellow polyhedron above the side chain of Phe331 illustrated that increased steric bulk nearby would decrease inhibitory activity. A small region of red contours near the carbonyl group suggested that more negative substituents would be favorable in these regions for improving inhibitory potencies. This prediction is consistent with the observations that the hydrogen bond can be built between the carbonyl group and the NH group of Phe288. The blue contour around the benzene ring of Tyr330 showed that the protonated nitrogen could interact with the side chain of Tyr330 through the cation–π interaction. Another blue contour near the phenol group of Tyr334 demonstrated that adding some positive groups around there would be beneficial to biological activity. The positive group there could interact with the carboxyl group of Asp72 through electrostatic forces.
The steric and electrostatic fields of CoMSIA, as shown in Figure 6A, were generally in accordance with the field distribution of the CoMFA maps (Figure 5). Besides the structural features already mentioned in the CoMFA steric field analysis (Figure 6A), there was a small, yellow polyhedron below the side chain of Tyr334, which illustrated that the bulky substituent in this area would be unfavorable to biological activity. At the midway of the deep gorge, the aromatic side chains of Tyr330 and Tyr121 formed a bottleneck that was large enough to only permit a water molecule to pass through[3]. The residues Tyr121, Phe290, Tyr330, Phe331, and Tyr334 in the binding pocket of AChE were in the distance of less than 3.0 Å to the benzene moiety of the inhibitor, as shown in Figure 6A[34]. Therefore, this area must be very constricted and can not be allowed to add bulky groups.
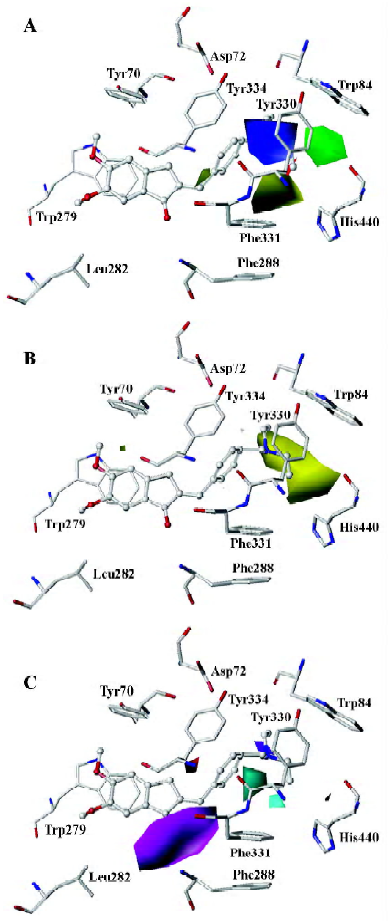
Hydrophobic interactions from CoMSIA As for the hydrophobic interaction, CoMSIA could illustrate clearly the hydrophobic interaction between the 1-indanone derivatives and AChE (Figure 6B). A big, yellow contour near the diethylamine group suggested that more hydrophobic groups could increase biological activity dramatically (Figure 6B), which is why the activity of the inhibitors had the order of 37<39<41<43<45. The diethylamine group of compound 31 was much more hydrophobic than the dimethylamine group of compound 26, which could account for the biological activity of compound 31 being much higher than that of compound 26.
Hydrogen bond interactions from CoMSIA As shown in Figure 6C, the hydrogen bond donor and acceptor maps of CoMSIA were in agreement with the inhibitor-protein binding model. The carbonyl group could form a strong hydrogen bond with the NH group of Phe288. As donepezil interacted with the AChE, the charged nitrogen might make an in-line hydrogen bond with WAT1159, which in turn would form H bonds with hydroxyl group of Tyr121, WAT 1158, and WAT 1160, similarly; the benzyl group makes a classic aromatic hydrogen bond (H-bond) with a water molecule (WAT 1160)[4]. That explains why there are 2 H-bond-donor-favored areas around the protonated nitrogen. Adding a hydrogen acceptor group to the 2-position substitutions on the diethylamine group would be detrimental to biological activity, as shown in Figure 6C.
Implication for new inhibitor design Combining all the above mapping information, it was obvious that a more hydrophobic and bulky group with a highly positive charge to replace the small protonated nitrogen moiety could increase the binding affinity through hydrophobic, Van der Waals, and cation–π forces with Trp84, Tyr330, and His440. For example, a phenyl ring linking to the protonated nitrogen might increase the biological activity of 2-substituted 1-indanone derivatives.
In conclusion, molecule docking and 3D-QSAR studies were carried out, not only to explore the interaction mechanism between 1-indanone derivatives and AChE, but also to construct highly accurate and predictive 3D-QSAR models to design new AChE inhibitors for the treatment of AD. The modeling results provided a satisfactory explanation for the binding interaction of the 1-indanone compounds with AChE. The reliability of the models was further verified by the inhibitors in the external test set and the leave-4-out cross-validation method. The 3D-QSAR results suggested that some important Van der Waals, electrostatic, hydrophobic, and H-bond forces contributed to raise the bioactivity of compounds. Thus, useful clues could be obtained from the models, which would be helpful for designing novel inhibitors of AChE with high potency and specific activity.
References
- Lahiri DK, Farlow MR, Greig NH, Sambamurti K. Current drug targets for Alzheimer’s disease treatment. Drug Dev Res 2002;56:267-81.
- Silman I, Sussman JL. Acetylcholinesterase: ‘classical’ and ‘non-classical’ functions andpharmacology. Curr Opin Pharmacol 2005;5:293-302.
- Raves ML, Harel M, Pang YP, Silman I, Kozikowski AP, Sussman JL. Structure of acetylcholinesterase complexed with the nootropic alkaloid, (–)-huperzine A. Nat Struct Biol 1997;4:57-63.
- Kryger G, Silman I, Sussman JL. Structure of acetylcholinesterase complexed with E2020 (Aricept): implications for the design of new anti-Alzheimer drugs. Struct 1999;7:297-307.
- Harel M, Schalk I, Ehret-Sabatier L, Bouet F, Goeldner M, Hirth C, et al. Quaternary ligand binding to aromatic residues in the active-site gorge of acetylcholinesterase. Proc Natl Acad Sci USA 1993;90:9031-5.
- Harel M, Quinn DM, Nair HK, Silman I, Sussman JL. The X-ray structure of a transition state analog complex reveals the molecular origins of the catalytic power and substrate specificity of acetylcholinesterase. J Am Chem Soc 1996;118:2340-6.
- Greenblatt HM, Kryger G, Lewis T, Silman I, Sussman JL. Structure of acetylcholinesterase complexed with (–)-galanthamine at 2.3 Å resolution. FEBS Lett 1999;463:321-6.
- Ravelli RB, Raves ML, Ren Z, Bourgeois D, Roth M, Kroon J, et al. Static Laue diffraction studies on acetylcholinesterase. Acta Crystallogr D Biol Crystallogr 1998;54:1359-66.
- Inestrosa NC, Alvarez A, Perez CA, Moreno RD, Vicente M, Linker C, et al. Acetylcholinesterase accelerates assembly of amyloid-beta-peptides into Alzheimer’s fibrils: possible role of the peripheral site of the enzyme. Neuron 1996;16:881-91.
- Bartolini M, Bertucci C, Cavrini V, Andrisano V. Beta-amyloid aggregation induced by human acetylcholinesterase: inhibition studies. Biochem Pharmacol 2003;65:407-16.
- Munoz-Muriedas J, Lopez JM, Orozco M, Luque FJ. Molecular modelling approaches to the design of acetylcholinesterase inhibitors: new challenges for the treatment of Alzheimer’s disease. Curr Pharm Des 2004;10:3131-40.
- Du DM, Carlier PR. Development of bivalent acetylcholinesterase inhibitors as potential therapeutic drugs for Alzheimer’s disease. Curr Pharm Des 2004;10:3141-56.
- Soreq H, Seidman S. Acetylcholinesterase-new roles for an old actor. Nat Rev Neurosci 2001;2:294-302.
- Sheng R, Lin X, Li J, Jiang Y, Shang Z, Hu Y. Design, synthesis, and evaluation of 2-phenoxy-indan-1-one derivatives as acetylcholinesterase inhibitors. Bioorg Med Chem Lett 2005;15:3834-7.
- Sheng R, Lin X, Li J, Jiang Y, Hu Y. Design, synthesis, and evaluation of 2-benzylidene-indan-1-one and 2-benzyl-indan-1-one derivatives as acetylcholinesterase inhibitors. In: Lee HZ, Hu YZ, editors. Proceedings of the 7th China–Japan Joint Symposium on Drug Design and Development; 22–26 Sep 2005, Hangzhou, China. Asian Federation of Medicinal Chemistry. Hangzhou: China; 2005. p31−p33.
- Akula N, Lecanu L, Greeson J, Papadopoulos V. 3D QSAR studies of AChE inhibitors based on molecular docking scores and CoMFA. Bioorg Med Chem Letter 2006;16:6277-80.
- SYBYL (Computer program). Version 7.0. St Louis, MO: Tripos Associates; 2004.
- Clark M, Cramer RDI, van Opdenbosch N. Validation of the general purpose Tripos 5.2 force field. J Comput Chem 1989;10:982-1012.
- Gasteiger J, Marsili M. Iterative partial equalization of orbital electronegativity: a rapid access to atomic charges. Tetrahedron 1980;36:3219-28.
- ACD/Labs Online (I-Lab), pKa caculation. [cited 2006 Aug 6]. Available from URL: http://ilab.acdlabs.com/
- Gilson MK, Straatsma TP, McCammon JA, Ripoll DR, Faerman CH, Axelsen PH, et al. Open “back door” in a molecular dynamics simulation of acetylcholinesterase. Science 1994;263:1276-8.
- Wlodek ST, Antosiewicz J, McCammon JA. Prediction of titration properties of structures of a protein derived from molecular dynamics trajectories. Protein Sci 1997;6:373-82.
- Weiner SJ, Kollman PA, Case DA, Singh C, Ghio G, Alagona S, et al. A new force field for molecular mechanical simulation of nucleic acids and proteins. J Am Chem Soc 1984;106:765-84.
- Jones G, Willett P, Glen RC, Leach AR, Taylor R. Development and validation of a genetic algorithm for flexible docking. J Mol Biol 1997;267:727-48.
- Eldridge MD, Murray CW, Auton TR, Paolini GV, Mee RP. Empirical scoring functions: I. The development of a fast empirical scoring function to estimate the binding affinity of ligands in receptor complexes. J Comput Aided Mol Des 1997;11:425-45.
- Guo J, Hurley MM, Wright JB, Lushington GH. A docking score function for estimating ligand-protein interactions: application to acetylcholinesterase inhibition. J Med Chem 2004;47:5492-500.
- Cramer RD III, Patterson DE, Bunce JD. Comparative molecular field analysis. 1. Effect of shape on binding of steroids to carrier proteins. J Am Chem Soc 1988;110:5959-67.
- Klebe G, Abraham U, Mietzner T. Molecular similarity indices in a comparative analysis (CoMSIA) of drug molecules to correlate and predict their biological activity. J Med Chem 1994;37:4130.
- Xie Q, Tang Y, Li W, Wang XH, Qiu ZB. Investigation of the binding mode of (–)-meptazinol and bis-meptazinol derivatives on acetylcholinesterase using a molecular docking method. J Mol Model 2006;12:390-7.
- Sugimoto H, Yamanishi Y, Iimura Y, Kawakami Y. Donepezil hydrochloride (E2020) and other acetylcholinesterase inhibitors. Curr Med Chem 2000;7:303-39.
- Kaur J, Zhang MQ. Molecular modelling and QSAR of reversible acetylcholines-terase inhibitors. Curr Med Chem 2000;7:273-94.
- Golbraikh A, Tropsha A. Beware of q2! J Mol Graph Model 2002;20:269-76.
- Shi LM, Fang H, Tong W, Wu J, Perkins R, Blair RM, et al. QSAR models using a large diverse set of estrogens. J Chem Inf Comput Sci 2001;41:186-95.
- Zuo Z, Luo X, Zhu W, Shen J, Shen X, Jiang H, et al. Molecular docking and 3D-QSAR studies on the binding mechanism of statine-based peptidomimetics with beta-secretase. Bioorg Med Chem 2005;13:2121-31.
- Schrödinger (Computer program). Version 2006. Portland, OR: Schrödinger, LLC; 2006.